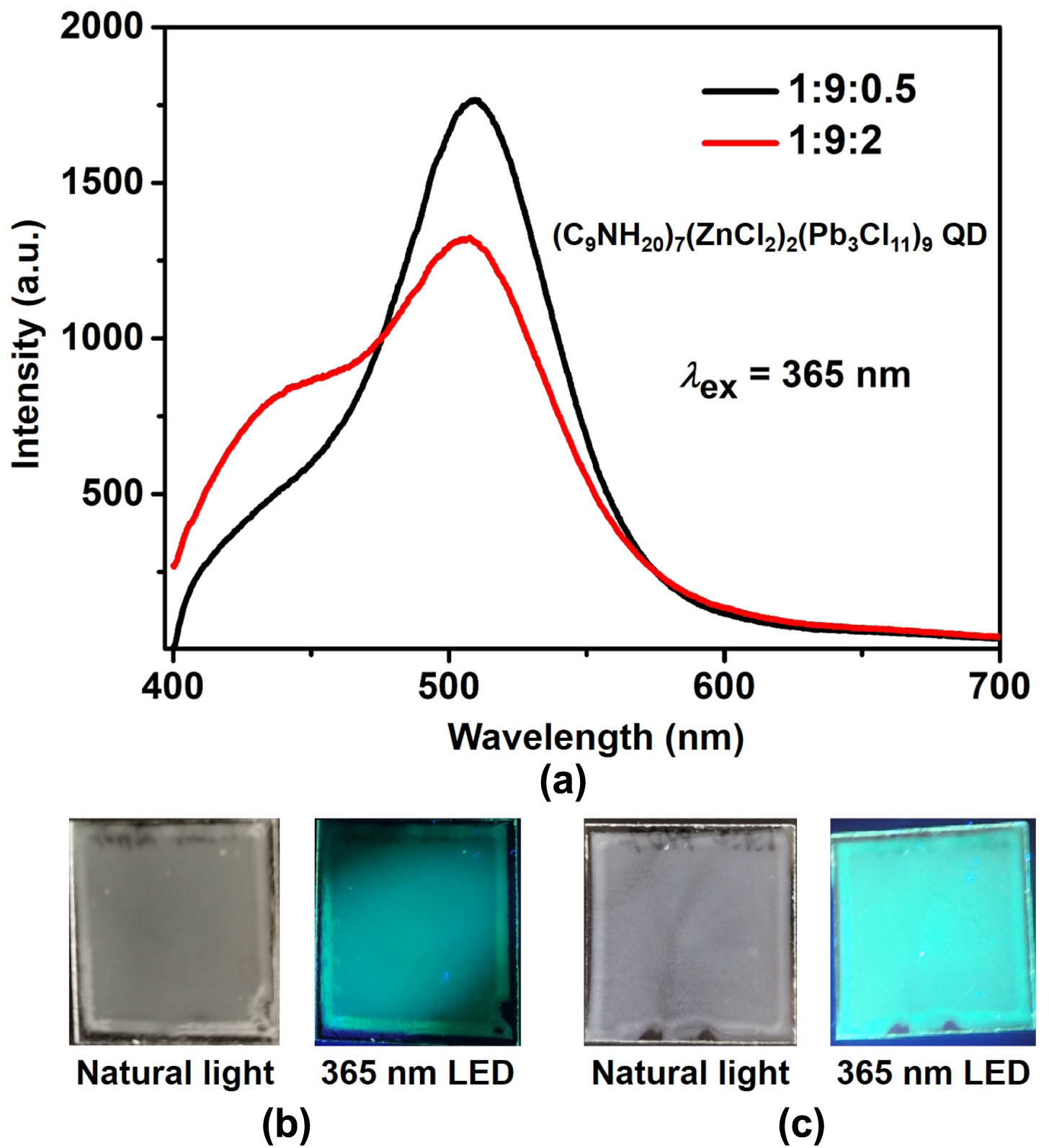
Author Affiliations
Abstract
1 Beijing National Research Center for Information Science and Technology (BNRist), Department of Electronic Engineering, Tsinghua University, Beijing 100084, China
2 China Mobile Research Institute, Beijing 100053, China
3 MIIT Key Laboratory for Low-dimensional Quantum Structure and Devices, School of Materials Sciences & Engineering, Beijing Institute of Technology, Beijing 100081, China
With the swift advancement of neural networks and their expanding applications in many fields, optical neural networks have gradually become a feasible alternative to electrical neural networks due to their parallelism, high speed, low latency, and power consumption. Nonetheless, optical nonlinearity is hard to realize in free-space optics, which restricts the potential of the architecture. To harness the benefits of optical parallelism while ensuring compatibility with natural light scenes, it becomes essential to implement two-dimensional spatial nonlinearity within an incoherent light environment. Here, we demonstrate a lensless opto-electrical neural network that incorporates optical nonlinearity, capable of performing convolution calculations and achieving nonlinear activation via a quantum dot film, all without an external power supply. Through simulation and experiments, the proposed nonlinear system can enhance the accuracy of image classification tasks, yielding a maximum improvement of 5.88% over linear models. The scheme shows a facile implementation of passive incoherent two-dimensional nonlinearities, paving the way for the applications of multilayer incoherent optical neural networks in the future.
Photonics Research
2024, 12(4): 682

Author Affiliations
Abstract
1 Tsinghua University, Beijing National Research Center for Information Science and Technology, Department of Electronic Engineering, Beijing, China
2 National University of Defense Technology, College of Advanced Interdisciplinary Studies, Hunan Provincial Key Laboratory of Novel Nano-Optoelectronic Information Materials and Devices, Changsha, China
On-chip diffractive optical neural networks (DONNs) bring the advantages of parallel processing and low energy consumption. However, an accurate representation of the optical field’s evolution in the structure cannot be provided using the previous diffraction-based analysis method. Moreover, the loss caused by the open boundaries poses challenges to applications. A multimode DONN architecture based on a more precise eigenmode analysis method is proposed. We have constructed a universal library of input, output, and metaline structures utilizing this method, and realized a multimode DONN composed of the structures from the library. On the designed multimode DONNs with only one layer of the metaline, the classification task of an Iris plants dataset is verified with an accuracy of 90% on the blind test dataset, and the performance of the one-bit binary adder task is also validated. Compared to the previous architectures, the multimode DONN exhibits a more compact design and higher energy efficiency.
optical computing mode multiplexing diffraction optical neural network Advanced Photonics Nexus
2024, 3(2): 026007
1 电子科技大学计算机科学与工程学院,四川 成都 611731
2 北京空间机电研究所,北京 100094
3 清华大学电子工程系,北京 100084
光学感前计算是一种在光电传感器前端的光学域进行信息计算处理的技术,包括编码压缩、全光智能推理等计算范式,具有传输即计算、结构即功能等显著特点,在卫星光学遥感领域有着广泛的应用前景。首先对用于感前计算的光场调制器件进行介绍,包括数字微镜器件(DMD)、液晶空间光调制器(LC-SLM)、衍射光学元件(DOE)及超表面等。然后分别梳理了感前编码压缩及全光智能推理的相关技术发展,在此基础上,着重讨论光学感前计算在卫星遥感领域的应用途径和未来发展趋势。
光学感前计算 编码压缩 全光智能推理 卫星遥感 激光与光电子学进展
2024, 61(2): 0211030
1 国防科技大学前沿交叉学科学院,湖南 长沙 410073
2 清华大学电子工程系,北京 100084
3 北京信息科学与技术国家研究中心,北京 100084
4 国防科技大学新型纳米光电信息材料与器件湖南省重点实验室,湖南 长沙 410073
5 国防科技大学南湖之光实验室,湖南 长沙 410073
光学神经网络是区别于冯·诺依曼计算架构的一种高性能新型计算范式,具有低延时、低功耗、大带宽以及并行信号处理等优势。片上集成是光学神经网络微型化发展的一种典型方式,近年来片上集成光学神经网络获得了学术界及工业界的广泛关注。对基于不同计算单元结构的片上集成光学神经网络的相关研究工作进行了梳理,并分析了其设计原理、实现方法及系统架构特征。同时结合国内外最新研究进展,进一步分析了片上集成光学神经网络在计算单元大规模拓展、可重构、非线性运算和实用化等方面面临的挑战及其未来发展趋势。
集成光学 光计算 光学神经网络 芯片 人工智能
1 联合微电子中心有限责任公司, 重庆 401332
2 电子科技大学 电子薄膜与集成器件国家重点实验室, 成都 610054
3 模拟集成电路国家级重点实验室, 重庆 400060
基于0.13 μm SiGe BiCMOS工艺,设计了一种线性驱动电路。该电路具有高速和大摆幅的优势,能线性驱动行波马赫-曾德尔调制器(TW-MZM),可满足光通信系统100 Gbit/s单通道的应用需求。驱动电路包括连续时间线性均衡(CTLE)电路、可变增益放大(VGA)电路和基于Cascode结构改进优化的输出级电路,实现了增益可调,且避免发生由较大输出摆幅导致的晶体管击穿。仿真结果表明,电路的-3 dB带宽为43 GHz,其增益在15~25 dB内可调。在56 Gbaud NRZ/PAM4的输入信号下,测得的眼图形状良好,差分输出摆幅峰-峰值达4 V,电路整体功耗为1.02 W,面积为0.33 mm2。
线性驱动电路 超高带宽 增益可调 大摆幅 linear driver ultra-high bandwidth adjustable gain large swing

Author Affiliations
Abstract
Beijing National Research Center for Information Science and Technology, Department of Electronic Engineering, Tsinghua University, Beijing 100084, China
Ever-growing deep-learning technologies are making revolutionary changes for modern life. However, conventional computing architectures are designed to process sequential and digital programs but are burdened with performing massive parallel and adaptive deep-learning applications. Photonic integrated circuits provide an efficient approach to mitigate bandwidth limitations and the power-wall brought on by its electronic counterparts, showing great potential in ultrafast and energy-free high-performance computation. Here, we propose an optical computing architecture enabled by on-chip diffraction to implement convolutional acceleration, termed “optical convolution unit” (OCU). We demonstrate that any real-valued convolution kernels can be exploited by the OCU with a prominent computational throughput boosting via the concept of structral reparameterization. With the OCU as the fundamental unit, we build an optical convolutional neural network (oCNN) to implement two popular deep learning tasks: classification and regression. For classification, Fashion Modified National Institute of Standards and Technology (Fashion-MNIST) and Canadian Institute for Advanced Research (CIFAR-4) data sets are tested with accuracies of 91.63% and 86.25%, respectively. For regression, we build an optical denoising convolutional neural network to handle Gaussian noise in gray-scale images with noise level , 15, and 20, resulting in clean images with an average peak signal-to-noise ratio (PSNR) of 31.70, 29.39, and 27.72 dB, respectively. The proposed OCU presents remarkable performance of low energy consumption and high information density due to its fully passive nature and compact footprint, providing a parallel while lightweight solution for future compute-in-memory architecture to handle high dimensional tensors in deep learning.
Photonics Research
2023, 11(6): 1125
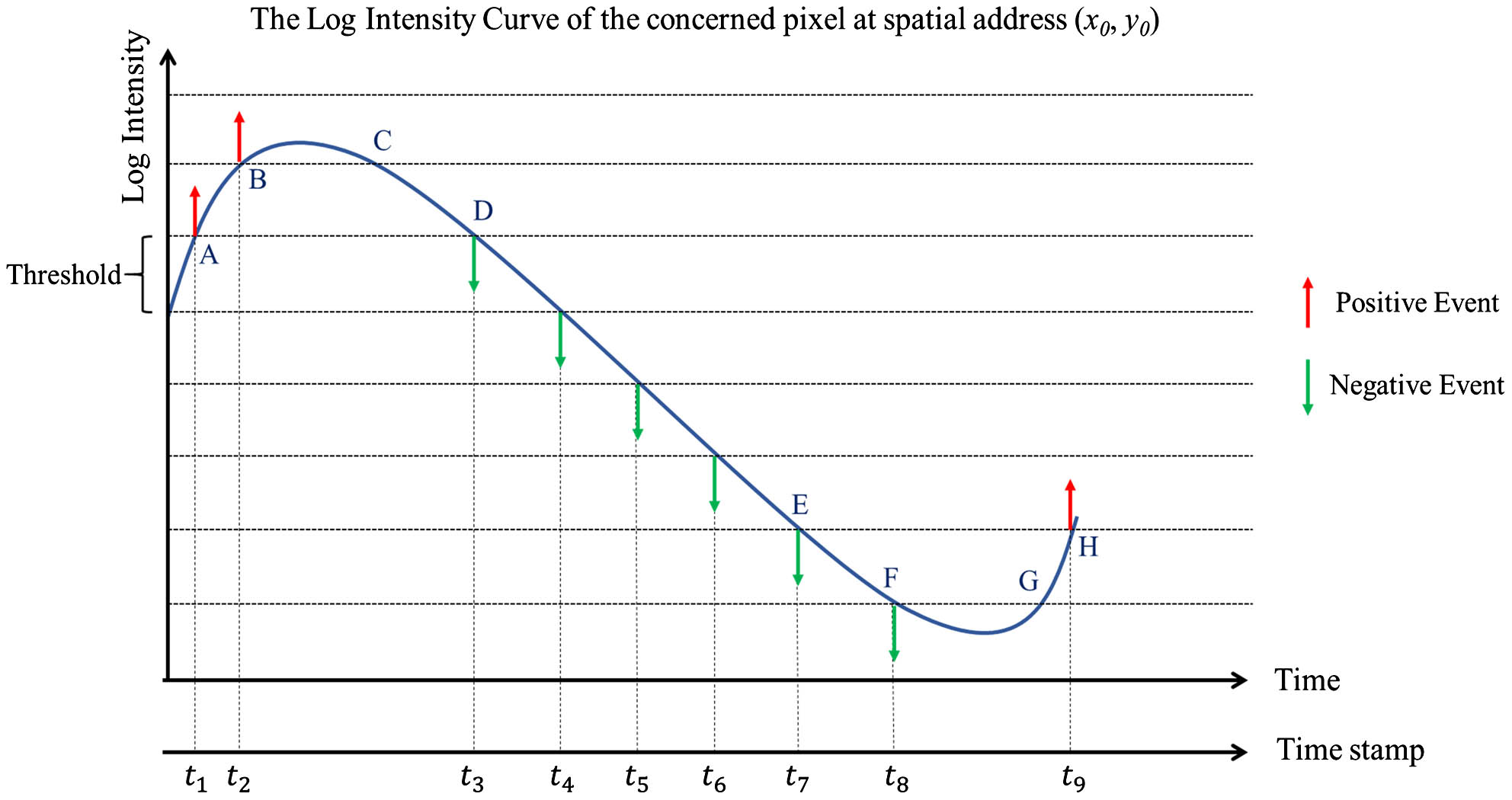
Author Affiliations
Abstract
1 Key Laboratory of Photoelectronic Imaging Technology and System of Ministry of Education of China, School of Optics and Photonics, Beijing Institute of Technology, Beijing 100081, China
2 Beijing National Research Center for Information Science and Technology (BNRist), Department of Electronic Engineering, Tsinghua University, Beijing 100084, China
Non-line-of-sight (NLOS) imaging is an emerging technique for detecting objects behind obstacles or around corners. Recent studies on passive NLOS mainly focus on steady-state measurement and reconstruction methods, which show limitations in recognition of moving targets. To the best of our knowledge, we propose a novel event-based passive NLOS imaging method. We acquire asynchronous event-based data of the diffusion spot on the relay surface, which contains detailed dynamic information of the NLOS target, and efficiently ease the degradation caused by target movement. In addition, we demonstrate the event-based cues based on the derivation of an event-NLOS forward model. Furthermore, we propose the first event-based NLOS imaging data set, EM-NLOS, and the movement feature is extracted by time-surface representation. We compare the reconstructions through event-based data with frame-based data. The event-based method performs well on peak signal-to-noise ratio and learned perceptual image patch similarity, which is 20% and 10% better than the frame-based method.
non-line-of-sight imaging event camera event-based representation Chinese Optics Letters
2023, 21(6): 061103
针对分布式群系统编队控制问题, 提出一种有向拓扑条件下的分布式编队控制方法。首先, 通过在控制协议中引入辅助函数, 利用变量代换, 将编队控制问题简化为自治系统的稳定性问题, 使得复杂的控制问题简单化。其次, 通过求解线性矩阵不等式设计控制增益矩阵, 形式简单, 求解复杂度低。然后借助李雅普诺夫方法分析系统的稳定性。仿真实验表明, 多无人机系统在分布式控制协议下, 能够有效实现编队跟踪控制。
多无人机系统 有向拓扑 编队跟踪 一致性 增益矩阵 multi-UAV systems directed topology formation tracking consensus gain matrix
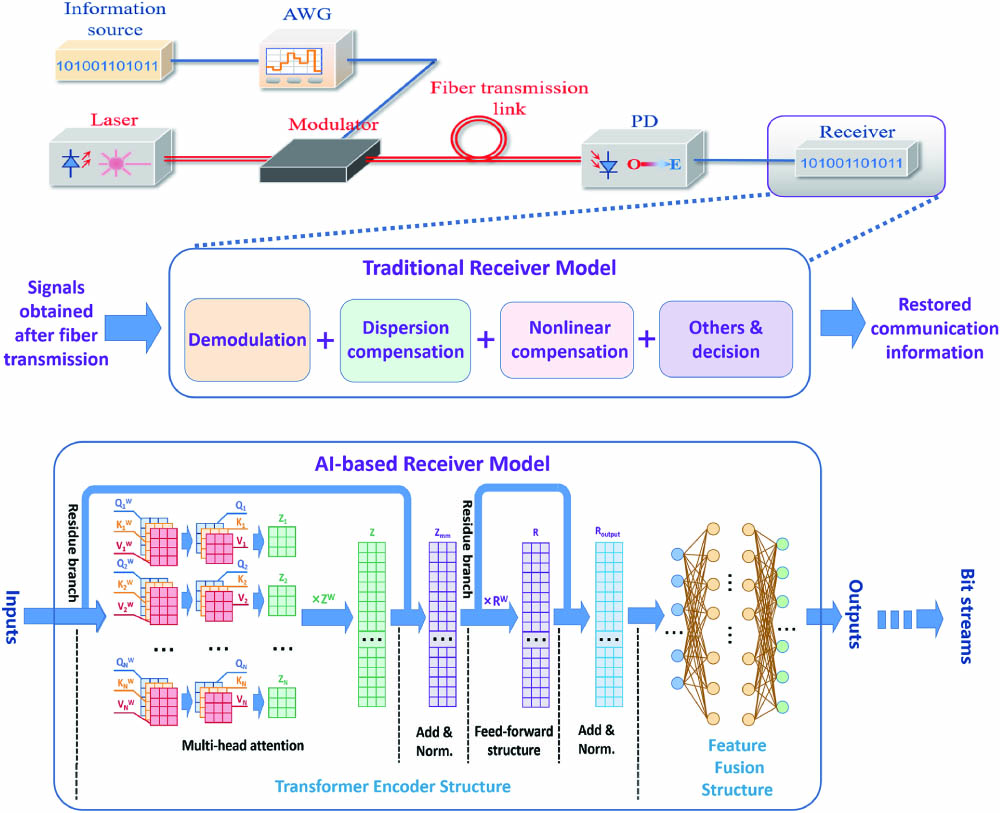
Author Affiliations
Abstract
1 Beijing National Research Center for Information Science and Technology (BNRist) and Department of Electronic Engineering, Tsinghua University, Beijing 100084, China
2 State Key Laboratory of Information Phonetics and Optical Communications, Beijing University of Post and Telecommunications, Beijing 100876, China
In this paper, an artificial-intelligence-based fiber communication receiver model is put forward. With the multi-head attention mechanism it contains, this model can extract crucial patterns and map the transmitted signals into the bit stream. Once appropriately trained, it can obtain the ability to restore the information from the signals whose transmission distances range from 0 to 100 km, signal-to-noise ratios range from 0 to 20 dB, modulation formats range from OOK to PAM4, and symbol rates range from 10 to 40 GBaud. The validity of the model is numerically demonstrated via MATLAB and Pytorch scenarios and compared with traditional communication receivers.
fiber receiver model neural networks multi-head attention mechanism Chinese Optics Letters
2023, 21(3): 030602

Author Affiliations
Abstract
1 Beijing National Research Center for Information Science and Technology (BNRist), Department of Electronic Engineering, Tsinghua University, Beijing 100084, China
2 State Key Laboratory of Advanced Optical Communications System and Networks, Department of Electronics, School of Electronics Engineering and Computer Science, Peking University, Beijing 100871, China
3 State Key Laboratory of Advanced Optical Communication Systems and Networks, Intelligent Microwave Lightwave Integration Innovation Center (imLic), Department of Electronic Engineering, Shanghai Jiao Tong University, Shanghai 200240, China
Microwave photonic receivers are a promising candidate in breaking the bandwidth limitation of traditional radio-frequency (RF) receivers. To further balance the performance superiority with the requirements regarding size, weight, and power consumption (SWaP), the implementation of integrated microwave photonic microsystems has been considered an upgrade path. However, up to now, to the best of our knowledge, chip-scale fully integrated microwave photonic receivers have not been reported due to the limitation of material platforms. In this paper, we report a fully integrated hybrid microwave photonic receiver (FIH-MWPR) obtained by comprising the indium phosphide (InP) laser chip and the monolithic silicon-on-insulator (SOI) photonic circuit into the same substrate based on the low-coupling-loss micro-optics method. Benefiting from the integration of all optoelectronic components, the packaged FIH-MWPR exhibits a compact volume of and low power consumption of 1.2 W. The FIH-MWPR supports a wide operation bandwidth from 2 to 18 GHz. Furthermore, its RF-link performance to down-convert the RF signals to the intermediate frequency is experimentally characterized by measuring the link gain, the noise figure, and the spurious-free dynamic range metrics across the whole operation frequency band. Moreover, we have utilized it as a de-chirp receiver to process the broadband linear frequency-modulated (LFM) radar echo signals at different frequency bands (S-, C-, X-, and Ku-bands) and successfully demonstrated its high-resolution-ranging capability. To the best of our knowledge, this is the first realization of a chip-scale broadband fully integrated microwave photonic receiver, which is expected to be an important step in demonstrating the feasibility of all-integrated microwave photonic microsystems oriented to miniaturized application scenarios.
Photonics Research
2022, 10(6): 06001472